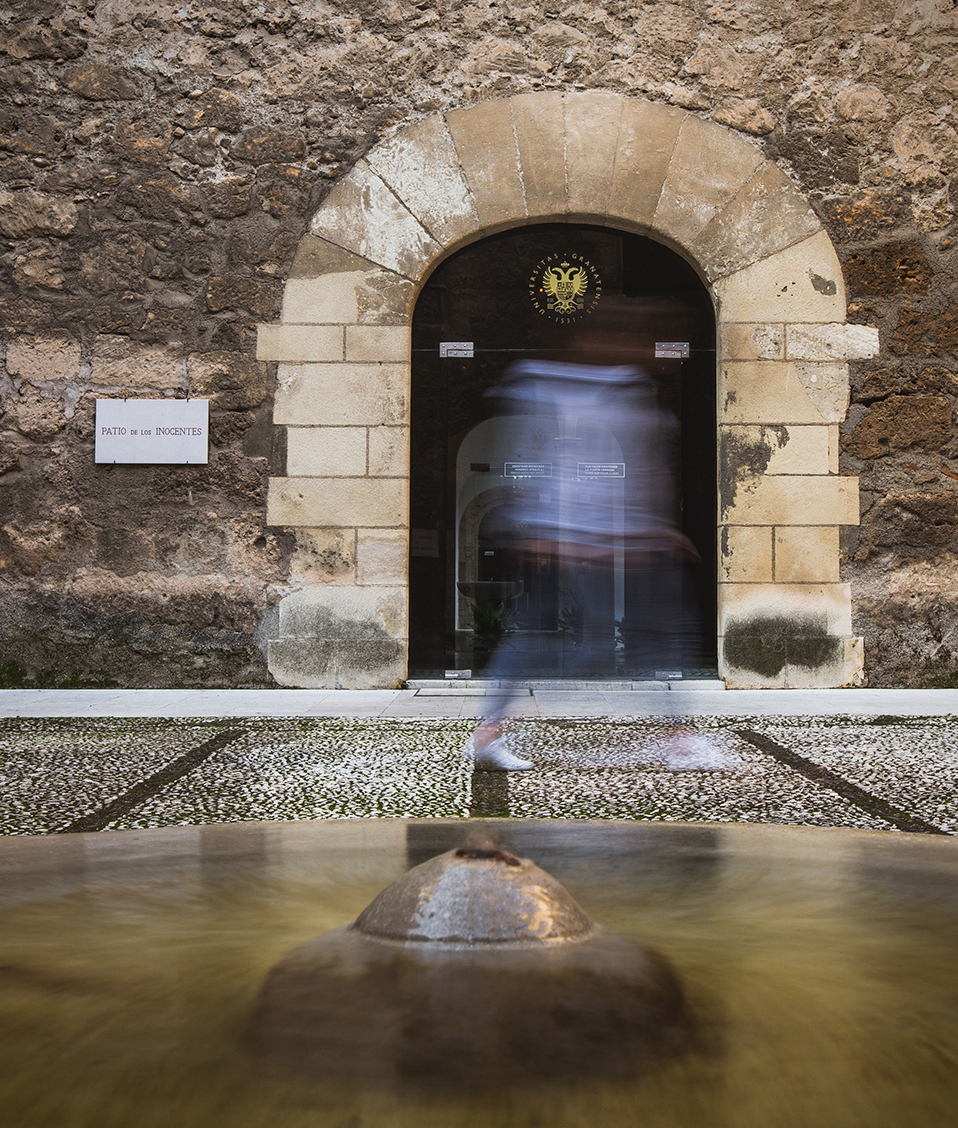
Jueves 24 de abril a las 12 pm en la Sala de Conferencias 1
“Recent developments in Linear Mixed-Effects Models” It turns out that we have all analyzed our data incorrectly, because we have usually failed to include by-stimulus random effects. When participants are exposed to multiple stimuli, it is not enough to treat only participants as a random factor (which is what we do in our standard ANOVAs), but we also have to treat stimuli as a random factor. Failure to do so increases the type-I error rate drastically, sometimes as high as 60%. Judd et al. (2012) were the first to raise awareness about this point. The problem: In order to treat both participants and stimuli as random factors, we have to run linear-mixed effects models, a rather complicated statistical procedure. Recent work by Barr et al. (2013) shows that many people run incorrect linear-mixed effects models. Many researchers include only by-stimulus random intercepts but fail to include by-stimulus random slopes. This leads once more to increased type-I error rates. The correct analytic procedure is to include both random intercepts and random slopes and, if the model doesn't converge, to take a number of “corrective actions” that can be ordered from yielding the most correct to the least correct estimates and that should be taken one by one (i.e., a corrective action further down on the list should be taken only if none of the previous actions fix the problem). On the list of corrective actions, the action “remove the random-intercept (but keep the random-slope)” comes much earlier than the action “remove the random-slope”. Removing the random slope is the very last thing we want to do, and should be done only in extreme cases